A conversation with Atif Syed on the sensitive side of robots
A conversation with Atif Syed on the sensitive side of robots
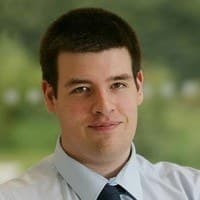
Technology and Innovation Officer
Wootzano was founded in 2018 after Atif Syed completed his PhD in Engineering and Electronics at the University of Edinburgh. With a focus on the synthesis of nanostructures, and the fabrication of flexible electronic substrates for biosensing applications, he wished to pursue the commercial applications of the technology he had developed.
Atif brought Wootzano to CPI to access the electronics equipment and expertise critical to enabling the scale-up of their flexible sensor technology. In just a few short years, he has built the company to the point where it has nine full time staff and has moved into a dedicated facility in the adjacent incubator space, while still using the openaccess clean rooms at CPI’s National Printable Electronics Centre. David Bird, Technology and Innovation Officer at CPI, caught up with Atif to find out what Wootzano is currently working on, their efforts in making a sensor skin for robots for automating picking and processing in Agritech.
Atif, what is the industrial impact of the system?
AS: The fresh produce industry was struggling before Brexit and COVID-19, there were always labour shortages ‘in the field’. For the packers, their biggest expense and pain is labour, both the cost, which can be 60% of their turnover, and finding staff. There are recruitment fees, and there is no guarantee that the people will be there the following day.
This is a big issue because margins are often very low and delaying deadlines for shipping to supermarkets or mis-packing leads to penalties. In this industry, 4% profit is a good year, and often can be 1 – 2%, so any additional admin costs can put a huge dent in profits. The pandemic is exceptional, but the robot system mitigates it to some degree because the robot substitutes the biggest expense, which is labour plus admin and overheads.
What is the robot system and how does it work?
AS: The core-essence of the system hardware for picking tomatoes and grapes is a commercially ready robotic system, compromised of a robot arm, an end-effector, and many of sensors, such as force, pressure-feedback, temperature and humidity. In development we have chemical sensors that can sense things such as the fruit’s ethylene signature, which we can use to indicate how long it will last before the onset of botrytis (grey mould), which gives confidence in the shelf-life of the produce.
There are some differences in the systems in that grape robot also has a snipper integrated to the gripper to cut the bunch from the vine and has more complex algorithms because it is aiming to cut bunches to a specific weight. The system is cost-effective for the grower because the cost and productivity of the robot is equivalent to 3 people over 3 shifts.
What else is needed; computers, software etc?
AS: The customer also gets a full computer system, which has a high-spec processor and graphic card, which are needed to run the LIDAR and stereo visual camera systems which lets the robot 15know where it is. The sensors are embedded in a compliant material so as not to damage the fruit, this will wear over time and so needs replacement. With the connection to cloud servers the robot can update its status, and we can ship replacement parts before they are needed so there is no down time
The sensors seem to be the critical part when compared to a robot that builds cars?
AS: Yes, we manufacture the sensors in CPI’s cleanroom, their unique property is their ability to send data continuously that can be fed into the machine learning module; this is where the ability to not only detect the force applied to the fruit to hold it without damaging it, but also to detect when the grip is slipping that gives these robots their remarkable abilities.
That must lead to a lot of data?
AS: With some measurements being every 10 milliseconds then there is a lot of data. We send this to the cloud where we use an AWS cluster of NVIDIA A100, which are £200,000 for one system, and we use 10 of them when establishing the models. Therefore, none of the machine learning is done on-site but having an engineer in the loop initially is critical. The type of machine learning (ML) we run is ‘supervised learning’ where when we collect the data and a ML engineer will update the model and then feed it back to the system so that the work becomes more efficient.
This contrast to ‘unsupervised ML’ where the algorithm makes up its own mind based on some seed parameters, so for example it would have to learn to pick up a tomato as well as how it should not do it, and so unsupervised ML will make mistakes many times before it is optimised. That would be very difficult to do on a production site, and so supervised ML is the best method.
Often a robot replacing a human job is not seen as a good thing, this is more positive though?
AS: In short, yes. Our systems will replace the hard laborious jobs. Fruit and veg pickers are subjected to gruelling, repetitive tasks, often having to endure the rapidly changing temperature conditions and long working hours. These systems will not only ensure that food supply for the masses is met but are consistent in meeting the ever-growing needs of a rising population. By replacing these types of jobs with robotics, we are creating new jobs in the industry which will ensure better working conditions for human workers. Moreover, we have seen during the pandemic a desire to reduce contact between humans and food, even with the best intentions, it is a difficult environment to control, often needing hundreds of people
How does the customer benefit from the robot system?
AS: We run a subscription service for the machine learning that enables superior models and updates to be applied to the customers robot so that they benefit from efficiency gains, this also includes hardware upgrades so when the ethylene sensors are released then our current customers will get that automatically. The robot continuously learns from itself and other robots in the distributed cloud network.
This makes the robot perform the task better every day as the data is collected from the skin (such as force or pressure, direction of force, temperature and humidity, and chemical signatures), vision system and custom built electronics giving data on the movements and trajectory the robot is making. All of this data is sent every 1 – 10 ms. This improvement makes the customers feel like they get a new robot as these updates are sent over-the-air.

How do you adapt the robot system for new species?
AS: There is a hardware adaptation, such as the snips needed for the grape-harvesting robot, but then we have to create a model for each species. There is no previous data that we can build the model on, often we are collecting this type of data for the first time ever, but once the model is established it only has to deal with the random events. These include the environment around the robot, such as dust, people walking around, and how the surroundings change throughout the year.
Beyond its basic task there are multiple things that occur during a day, week or month that the robot must cope with, therefore we need to change the models to create some intuition in the robot. We always go with a safe approach; for example if the robot is unsure of the condition of the fruit that it is holding then it will put it on the side for a human to look at, and we then give it feedback to encourage the right behaviour.

What are the next applications?
AS: Our fruit and veg robots are designed to be versatile, the grippers and picking fingers are replaceable, and made by us, with some outsourcing of standardised components. This means we can adapt to other materials and markets. Tomatoes and grapes are similar, although you need to find stem snips for grapes, but next there are mangoes, avocado, kiwis, and vine-based products in the pipeline. The market for agricultural robots is massive, we are looking at a potential £300m market in 2 – 3 years if we can capture larger players who can justify robots.
We then expect economies of scale to help us bring the price of the robots down, and so in 5 years we can address the smaller packers. Another application is using end effectors for prosthetics so that amputees can feel what they are holding, including its temperature. We hope to reach the market with that in 3 – 4 years’ time. At that point the company would have to split into a group with subsidiaries. I envisage an agritech company and a prosthetics company, both supported by a research organisation for the sensors. Other obvious application such as environments that are hazardous to humans such as nuclear power plants or servicing offshore wind turbine may also require a dedicated business unit.
The potential customer base for any of this in the UK, EU or North America is huge due to the higher labour costs. If things go right, in next 3 years we will float the company. I expect the company will change when that happens, but in the meantime, there is still a lot to learn about fruit and veg!
Enjoyed this article? Keep reading more expert insights...
CPI ensures that great inventions gets the best opportunity to become a successfully marketed product or process. We provide industry-relevant expertise and assets, supporting proof of concept and scale up services for the development of your innovative products and processes.